- deep learning
- overfeat
- Optimizer
- object detection
- Convolution 종류
- image classification
- Weight initialization
- 딥러닝
- SPP-Net
- LeNet 구현
일 | 월 | 화 | 수 | 목 | 금 | 토 |
---|---|---|---|---|---|---|
1 | 2 | 3 | 4 | |||
5 | 6 | 7 | 8 | 9 | 10 | 11 |
12 | 13 | 14 | 15 | 16 | 17 | 18 |
19 | 20 | 21 | 22 | 23 | 24 | 25 |
26 | 27 | 28 | 29 | 30 | 31 |
- Today
- Total
목록분류 전체보기 (56)
I'm Lim
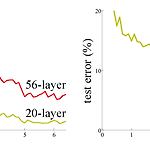
Paper He, Kaiming, et al. "Deep residual learning for image recognition." Proceedings of the IEEE conference on computer vision and pattern recognition. 2016. Abstract ResNet은 ILSVRC 2015 대회에서 우승한 모델로써 residual block이라는 이름의 모듈을 깊게 쌓은 모델이다. ResNet은 152개의 레이어 개수를 가진다. 이는 VGGNet보다 8배나 많은 레이어 개수고, 그럼에도 불구하고 ResNet은 lower complexity (파라미터 수가 적음)를 가진다. Introduction 1. degradation problem 기존의 모델들은 레이어를..
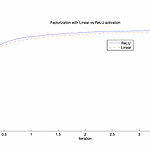
Paper Szegedy, Christian, et al. "Rethinking the inception architecture for computer vision." Proceedings of the IEEE conference on computer vision and pattern recognition. 2016. Abstract AlexNet에서부터 GoogLeNet에 이르기까지 딥러닝 모델링의 방향성은 모델의 크기와 컴퓨터 계산비용을 늘림으로써 성능을 향상시키는 것이었다. 하지만, 계산비용과 파라미터 개수를 줄이는 것은 모바일 환경에서의 사용과 빅데이터 상황에서 유용하게 작용할 것이다. 본 논문에서는 위를 근거로 컴퓨터 계산 비용을 줄이는 연구를 진행할 뿐만 아니라 Regularization을 적..
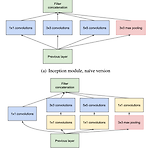
Paper Szegedy, Christian, et al. "Going deeper with convolutions." Proceedings of the IEEE conference on computer vision and pattern recognition. 2015. Abstract GoogLeNet은 ILSVRC 2014 우승을 차지한 모델로써 Inception 모듈을 통해 모델의 width를 늘림과 동시에 레이어의 depth를 22개까지 늘린 모델이다. Introduction GoogLeNet은 레이어를 22개를 쌓음으로써 ISLVRC 2012 우승 모델인 AlexNet의 성능을 제쳤다. 더욱 주목할만한 점은 AlexNet에 비해 12배나 적은 파라미터 수를 가진다는 것이다. Related Wor..
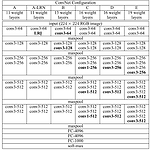
Paper Simonyan, Karen, and Andrew Zisserman. "Very deep convolutional networks for large-scale image recognition." arXiv preprint arXiv:1409.1556 (2014). Introduction VGGNet은 ILSVRC 2014에서 2등을 한 모델이다 (참고로, 1위는 GoogLeNet이다). VGG는 우수한 성능에도 그 구조가 매우 간단하다는 장점이 있다. 본 논문에서는 kernel size에 집중하기 보다는 모델의 깊이에 (레이어의 개수) 더욱 집중했다. Model Configuration - VGGNet은 모든 Conv 레이어의 kernel size를 3 x 3으로 고정시켰다. - 각 Conv ..
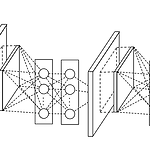
Paper Lin, Min, Qiang Chen, and Shuicheng Yan. "Network in network." arXiv preprint arXiv:1312.4400 (2013). Introduction 1. Generalized Linear Model Convolutional Neural Network는 Conv 레이어와 Pooling 레이어로 구성되어있다. CNN 필터는 feature map과 가중치 간의 선형 결합으로 표현된 후, non-linear function을 거친다. 이는 Generalized Linear Model (GLM) 과 유사한 방식이다. 따라서, 논문에서는 CNN 필터가 GLM 모델이라고 하였다. 2. Network In Network GLM은 latent space..
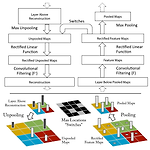
1. Paper Zeiler, Matthew D., and Rob Fergus. "Visualizing and understanding convolutional networks." European conference on computer vision. Springer, Cham, 2014. 2. Introduction 이 논문은 ILSVRC 2012 우승을 차지한 AlexNet 모델을 분석합니다. AlexNet 모델의 성능이 굉장히 좋은 것은 사실이지만, 왜 그런지에 대한 설명은 없고, 그렇기 때문에 성능 개선시킬 수 있는지도 알 수 없습니다. 이 논문에서는 그 이유에 대해 분석하고, 모델을 개선시킴으로써 AlexNet보다 우수한 성능을 달성했습니다. 3. Visualization with a Decon..
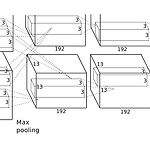
1. Paper Krizhevsky, Alex, Ilya Sutskever, and Geoffrey E. Hinton. "Imagenet classification with deep convolutional neural networks." Communications of the ACM 60.6 (2017): 84-90. 2. Introduction AlexNet은 ILSVRC-2012 contest에서 기존의 머신러닝 기반 모델들을 모두 제치고, 1등을 차지한 최초의 딥러닝 모델입니다. 또한, 전년 우승작의 Top-5 error rate를 10%이상 감소시켰습니다. 3. ImageNet Dataset 1 ) Resize AlexNet은 ImageNet 데이터셋을 이용하여 모델 학습을 진행하였습니다. 이 ..
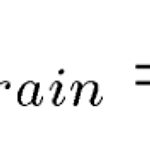
1. Paper LeCun, Yann, et al. "Gradient-based learning applied to document recognition." Proceedings of the IEEE 86.11 (1998): 2278-2324. 2. 전통적인 패턴 인식 1 ) feature extract를 위해서는 사전지식과 업무를 구체적으로 알아야합니다. 극단적인 예시로 모든 개는 수염이 없고 고양이는 수염이 있다고 가정한다면, 수염의 여부로 개와 고양이를 100퍼센트 분류해낼 수 있습니다. 이는 적절한 인식 시스템이 만들어졌다고 볼 수 있습니다. 이 예시는 개와 고양이를 분류하는 업무라는 것을 구체적으로 파악하는 것과 개와 고양이를 분류하는 특징은 수염이라는 것을 파악해야 올바른 인식 시스템을 만들 ..